Automated Insect Monitoring
Empowering local communities to protect and conserve biodiversity
Photo by Michael Bunsen
What is Automated Monitoring of Insects (AMI)?
AMI is an international consortium of experts developing non-lethal, end-to-end monitoring solutions for automated insect detection and species classification that leverage artificial intelligence (AI). Through technologies such as high-resolution cameras, low-cost sensors, and AI-based processing methods, AMI aims to make insect monitoring less labour-intensive, more user-friendly, and more effective at determining the state of insect populations across the globe. AMI strives to foster partnerships and knowledge-sharing to maximize the impact of monitoring and conservation efforts.
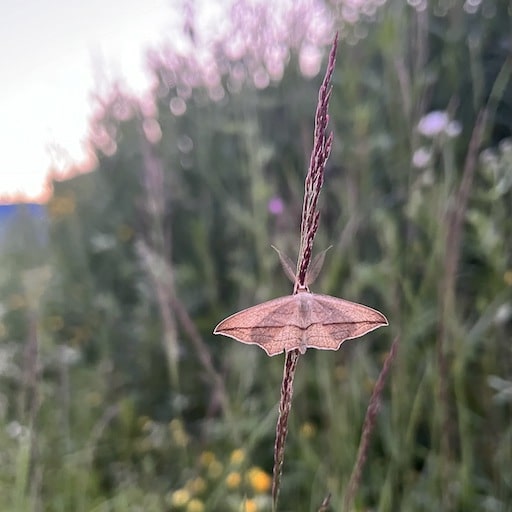
Photo by Anna Viklund
Why AMI is Needed
There are over a million known species of insects on Earth, representing nearly one half of all known organisms. Insects are instrumental to ecosystem functioning and provide services that are critical for humans, such as pollination and the control of pests and disease vectors. In many areas, the populations of a wide array of insect species are declining in abundance and diversity due to multiple stressors including climate change and human augmented landscapes. These changes raise concerns about the knock-on impact on ecosystem health and potential global ecological and economic consequences. Still, data on insect populations remains sparse. It is challenging and resource-intensive to collect and process data as well as to train subject matter experts to support the study and conservation of insects. There is therefore a worldwide need for robust, cost-effective monitoring methods to fill the data gap in a way that minimizes bias and maximizes the quantity and quality of data collected. AMI addresses this gap and enables scientists to track or predict insect biodiversity loss and mitigate its impacts on ecosystem functioning, human health, livelihoods, and economies. AMI currently focuses on the monitoring of one of the most biodiverse groups of insects: moths.
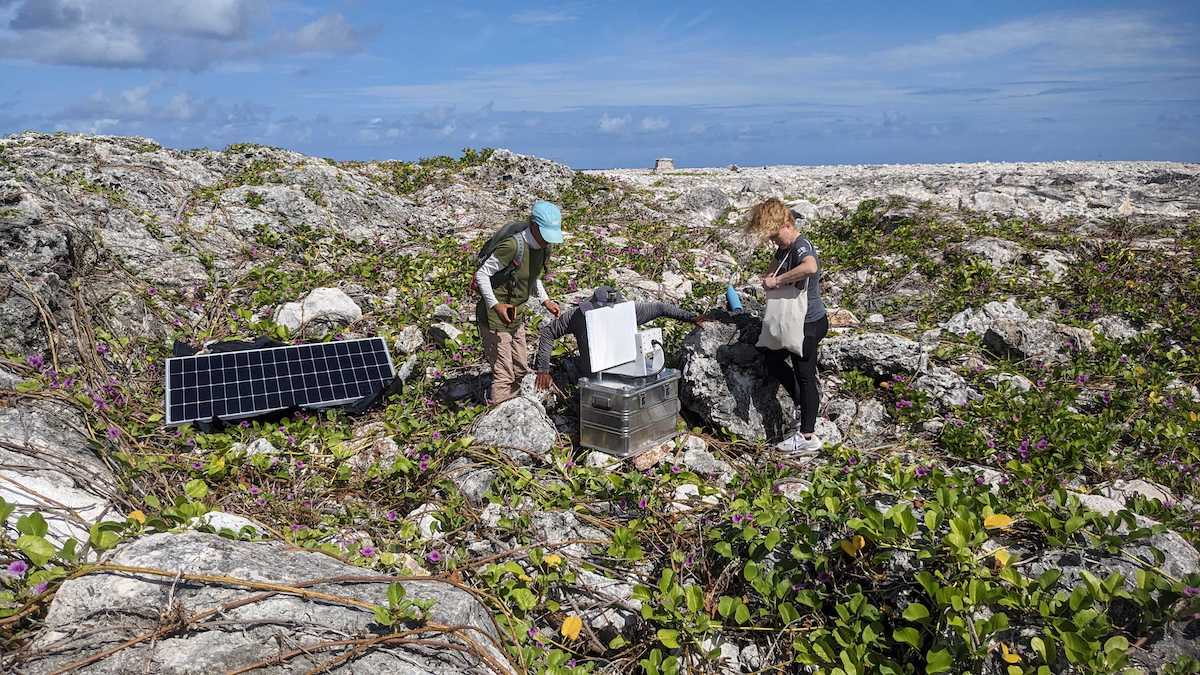
The UKCEH AMI System deployed at Anguilla. Photo by Tom August.
The AMI Initiatives
Collectively, the AMI Consortium offers a range of initiatives that cover the entire lifecycle of automated insect monitoring data: the hardware to capture images, the software to classify the images, and tools to support ecological insights.
Checkout the initiativesResources
The Antenna Data Platform is an interdisciplinary hub for automatically processing insect data at wide spatial, temporal and taxonomic scales. The platform uses state-of-the-art machine learning algorithms developed by Mila researchers to detect and classify insects in large volumes of images.
The AMI Dataset, consists of two parts: 1) AMI-GBIF, a dataset of ∼2.5M human-captured insect images curated from citizen science platforms and museum collections, 2) AMI-Traps, an expert-annotated dataset of 2,893 insect camera trap images (representing 52,948 labeled insects) collected from a global network of automated camera traps, designed to test in-the-wild performance.
The UKCEH AMI System consists of UV and white lights for attracting moths, high-capacity data storage to collate images over long sampling periods, battery and solar power to allow for deployment away from mains power, and customisable sampling schedules. The images it collects can be processed through one’s own workflow or using the AMI Data Platform.
Academic Publications
We present a portable computer vision system capable of attracting and detecting live insects. More specifically, the paper proposes detection and classification of species by recording images of live individuals attracted to a light trap. An Automated Moth Trap (AMT) with multiple light sources and a camera was designed to attract and monitor live insects during twilight and night hours. A computer vision algorithm referred to as Moth Classification and Counting (MCC), based on deep learning analysis of the captured images, tracked and counted the number of insects and identified moth species.
Insect Identification in the Wild: The AMI Dataset
We provide the first large-scale machine learning benchmarks for fine-grained insect recognition, designed to match real-world tasks faced by ecologists.
Towards a standardized framework for AI-assisted, image-based monitoring of nocturnal insects.
We describe computer vision approaches for the detection, tracking and classification of insects, including models built from existing aggregations of labelled insect images. We propose ten priorities towards a step-change in automated monitoring of nocturnal insects, a vital task in the face of rapid biodiversity loss from global threats.
Media
Un algorithme au secours des insectes | Découverte
Les populations d'insectes s'effondrent à un rythme sans précédent, et il manque de scientifiques pour documenter ce déclin.
Radio Canada